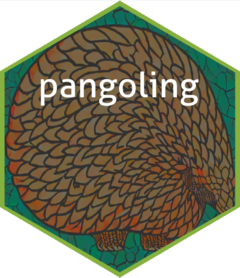
Get the possible tokens and their log probabilities for each mask in a sentence
Source:R/tr_masked.R
masked_tokens_pred_tbl.Rd
For each mask, indicated with [MASK]
, in a sentence, get the possible
tokens and their predictability (by default the natural logarithm of the
word probability) using a masked transformer.
Arguments
- masked_sentences
Masked sentences.
- log.p
Base of the logarithm used for the output predictability values. If
TRUE
(default), the natural logarithm (base e) is used. IfFALSE
, the raw probabilities are returned. Alternatively,log.p
can be set to a numeric value specifying the base of the logarithm (e.g.,2
for base-2 logarithms). To get surprisal in bits (rather than predictability), setlog.p = 1/2
.- model
Name of a pre-trained model or folder. One should be able to use models based on "bert". See hugging face website.
- checkpoint
Folder of a checkpoint.
- add_special_tokens
Whether to include special tokens. It has the same default as the AutoTokenizer method in Python.
- config_model
List with other arguments that control how the model from Hugging Face is accessed.
- config_tokenizer
List with other arguments that control how the tokenizer from Hugging Face is accessed.
Value
A table with the masked sentences, the tokens (token
),
predictability (pred
), and the respective mask number (mask_n
).
Details
A masked language model (also called BERT-like, or encoder model) is a type of large language model that can be used to predict the content of a mask in a sentence.
If not specified, the masked model that will be used is the one set in
specified in the global option pangoling.masked.default
, this can be
accessed via getOption("pangoling.masked.default")
(by default
"bert-base-uncased"). To change the default option
use options(pangoling.masked.default = "newmaskedmodel")
.
A list of possible masked can be found in Hugging Face website
Using the config_model
and config_tokenizer
arguments, it's possible to
control how the model and tokenizer from Hugging Face is accessed, see the
python method
from_pretrained
for details. In case of errors check the status of
https://status.huggingface.co/
More examples
See the online article in pangoling website for more examples.
See also
Other masked model functions:
masked_targets_pred()
Examples
masked_tokens_pred_tbl("The [MASK] doesn't fall far from the tree.",
model = "bert-base-uncased"
)
#> Processing using masked model 'bert-base-uncased/' ...
#> # A tidytable: 30,522 × 4
#> masked_sentence token pred mask_n
#> <chr> <chr> <dbl> <int>
#> 1 The [MASK] doesn't fall far from the tree. snow -3.12 1
#> 2 The [MASK] doesn't fall far from the tree. rock -3.45 1
#> 3 The [MASK] doesn't fall far from the tree. stone -3.77 1
#> 4 The [MASK] doesn't fall far from the tree. girl -3.85 1
#> 5 The [MASK] doesn't fall far from the tree. sun -3.99 1
#> 6 The [MASK] doesn't fall far from the tree. tree -3.99 1
#> 7 The [MASK] doesn't fall far from the tree. branch -4.09 1
#> 8 The [MASK] doesn't fall far from the tree. body -4.12 1
#> 9 The [MASK] doesn't fall far from the tree. light -4.43 1
#> 10 The [MASK] doesn't fall far from the tree. water -4.44 1
#> # ℹ 30,512 more rows