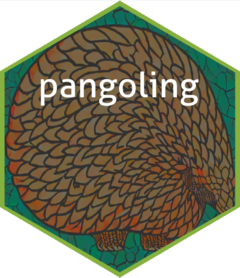
Get the log probability of each element of a vector of words (or phrases) using a causal transformer
Source:R/tr_causal.R
causal_lp.Rd
Get the log probability of each element of a vector of words (or phrases) using a causal transformer model. See the online article in pangoling website for more examples.
Arguments
- x
Vector of words, phrases or texts.
- by
Vector that indicates how the text should be split.
- l_contexts
Left context for each word in
x
. Ifl_contexts
is used,by
is ignored. Setby = NULL
to avoid a message notifying that.- ignore_regex
Can ignore certain characters when calculates the log probabilities. For example
^[[:punct:]]$
will ignore all punctuation that stands alone in a token.- model
Name of a pre-trained model or folder.
- checkpoint
Folder of a checkpoint.
- add_special_tokens
Whether to include special tokens. It has the same default as the AutoTokenizer method in Python.
- config_model
List with other arguments that control how the model from Hugging Face is accessed.
- config_tokenizer
List with other arguments that control how the tokenizer from Hugging Face is accessed.
- batch_size
Maximum size of the batch. Larges batches speedup processing but take more memory.
- ...
not in use.
Details
A causal language model (also called GPT-like, auto-regressive, or decoder model) is a type of large language model usually used for text-generation that can predict the next word (or more accurately in fact token) based on a preceding context.
If not specified, the causal model that will be used is the one set in
specified in the global option pangoling.causal.default
, this can be
accessed via getOption("pangoling.causal.default")
(by default
"gpt2"). To change the default option
use options(pangoling.causal.default = "newcausalmodel")
.
A list of possible causal models can be found in Hugging Face website.
Using the config_model
and config_tokenizer
arguments, it's possible to
control how the model and tokenizer from Hugging Face is accessed, see the
Python method
from_pretrained
for details.
In case of errors when a new model is run, check the status of https://status.huggingface.co/
More examples
See the online article in pangoling website for more examples.
See also
Other causal model functions:
causal_config()
,
causal_lp_mats()
,
causal_next_tokens_tbl()
,
causal_preload()
,
causal_tokens_lp_tbl()
Examples
if (FALSE) { # interactive()
causal_lp(
x = c("The", "apple", "doesn't", "fall", "far", "from", "the", "tree."),
model = "gpt2"
)
causal_lp(
x = "tree.",
l_contexts = "The apple doesn't fall far from the tree.",
by = NULL, # it's ignored anyways
model = "gpt2"
)
}